NewsJul | 18 | 2023
Research Spotlight: Leveraging Self-Supervised Learning and Physics Inspired Augmentations for Enhanced Liver Segmentation in Ultrasound Imaging
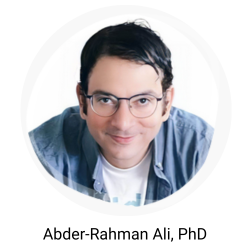
What Question Were You Investigating?
The main question this research aimed to investigate was whether a contrastive self-supervised learning approach (specifically employing SimCLR) combined with ENet, in conjunction with physics-inspired augmentations, could effectively improve liver segmentation in ultrasound images.
What Methods or Approach Did You Use?
We adopted a two-stage approach using a combination of self-supervised and supervised learning models. In the first stage, we used SimCLR, a contrastive self-supervised learning approach, trained on unannotated abdominal ultrasound images to learn the feature representations.
In the second stage, the model was fine-tuned using ENet on a smaller dataset of images with annotated liver segmentation masks. Furthermore, we introduced physics-inspired data augmentations, which included sector angle, penetration, gaussian blur, force control, and zoom, to better simulate the realities of ultrasound image scanning. Finally, the output of the model was refined using CascadePSP for improved segmentation results.
What Were Your Findings?
The proposed SimCLR+ENet approach consistently outperformed the U-Net model in liver segmentation tasks, particularly when trained on physics-augmented data. This superiority was evident in the higher Dice coefficient and lower Hausdorff distance achieved by our model, metrics that reflect better agreement with the ground truth and more accurate segmentation shapes, respectively. When we added CascadePSP for refinement, the results further improved, yielding outputs that closely mirrored the structure of the ground truth.
What Are the Clinical Implications?
The clinical implication of our work is its ability to enhance the accuracy and efficiency of diagnosing liver fibrosis. By improving the segmentation of the liver in ultrasound images, we can facilitate the use of non-invasive methods like Shear Wave Elastography (SWE) in identifying liver fibrosis, thereby reducing the need for invasive and potentially risky procedures like liver biopsies.
Improved accuracy in liver segmentation can lead to more accurate measurements of liver stiffness, which is a key biomarker for liver fibrosis. This means doctors can monitor the progression of liver disease more effectively, allowing for earlier intervention and treatment planning.
Furthermore, this method helps reduce reliance on heavily annotated data, which is often difficult to acquire in a clinical context, thus providing a scalable solution for wider clinical applications.
What Are the Next Steps?
The next steps with this research involve improving the quality of data used for training the downstream task by utilizing a data-centric AI approach, aiming to achieve better segmentation performance with less labeled data that has been systemically selected.
Additionally, we plan to explore the use of a vision transformer (ViT) as the backbone of SimCLR. This could potentially enhance the model's learning capability and thus its performance in liver segmentation tasks.
Paper Cited:
Ali, A.-R., Guo, P., & Samir, A. E. (2023). Liver Segmentation in Ultrasound Images Using Self-Supervised Learning with Physics-inspired Augmentation and Global-Local Refinement. Proceedings of the Canadian Conference on Artificial Intelligence. https://doi.org/10.21428/594757db.120db6ad
About the Massachusetts General Hospital
Massachusetts General Hospital, founded in 1811, is the original and largest teaching hospital of Harvard Medical School. The Mass General Research Institute conducts the largest hospital-based research program in the nation, with annual research operations of more than $1 billion and comprises more than 9,500 researchers working across more than 30 institutes, centers and departments. In August 2021, Mass General was named #5 in the U.S. News & World Report list of "America’s Best Hospitals." MGH is a founding member of the Mass General Brigham healthcare system.
Type
Centers and Departments
Topics
Check out the Mass General Research Institute blog
Bench Press highlights the groundbreaking research and boundary-pushing scientists working to improve human health and fight disease.
Support Research at Mass General
Your gift helps fund groundbreaking research aimed at understanding, treating and preventing human disease.