NewsJul | 18 | 2023
Research Spotlight: High-Accuracy Liver View Classification in Ultrasound Imaging Using Minimal Labeled Data
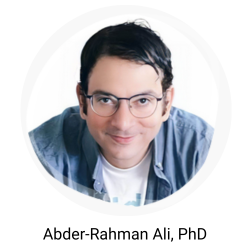
Abder-Rahman Ali, PhD, a Research Fellow in the Center for Ultrasound Research & Translation (CURT) at Massachusetts General Hospital, is the first author of a new study published in IEEE/CVF Conference on Computer Vision and Pattern Recognition (CVPR) Workshops, 2023, Self-Supervised Learning for Accurate Liver View Classification in Ultrasound Images with Minimal Labeled Data.
What Question Were You Investigating?
The primary question investigated in this research was: Can a two-stage approach combining self-supervised learning with logistic regression (termed SimCLR+LR) accurately classify liver views in abdominal ultrasound images, even when trained on a limited number of labeled samples, and does this approach outperform existing state-of-the-art models such as ResNet-18 and MLP-Mixer?
What Methods or Approach Did You Use?
The approach utilized was a two-stage method, named SimCLR+LR, which combined self-supervised and supervised learning techniques. Initially, a contrastive self-supervised learning method, SimCLR, was trained on a large dataset of unlabeled abdominal ultrasound images to extract feature representations. Following this, a supervised logistic regression model was used to fine-tune these feature representations, utilizing a substantially smaller labeled dataset of images classified into liver view classes. This approach aimed to classify the images into either "with liver" or "without liver" categories.
What Were Your Findings?
The findings of the study indicate that the SimCLR+LR method exhibited high classification accuracy even with a limited number of labeled images. When 50 images were used per class, liver view classification accuracies of 95.3%, 96%, and 99.3% were achieved with training epochs of 100, 500, and 1000, respectively. In a comparison against state-of-the-art models ResNet-18 and MLP-Mixer, SimCLR+LR demonstrated significantly better performance across different epochs. After training for 1000 epochs, SimCLR+LR achieved an impressive accuracy of 98.7%, substantially surpassing the 79.3% accuracy obtained by ResNet-18. For the individual liver view classes, SimCLR+LR achieved 95.1% accuracy for images categorized as "with liver", and 93.9% for "without liver", outperforming both ResNet-18 and MLP-Mixer. Remarkably, training SimCLR+LR with only one labeled image for each class yielded a performance similar to MLP-Mixer, which was trained on an entire dataset of 801 images.
What Are the Clinical Implications?
The use of the SimCLR+LR approach can potentially save time, reduce costs, and lessen reliance on human labor for labeling data, as it demonstrated high performance even with minimal labeled data. This methodology can be particularly useful when there is a need to select images of a specific organ from a larger dataset containing various organs, which can streamline the workflow in clinical settings. The approach could also be expanded to multiclass organ view classification, paving the way for more efficient and effective diagnostic procedures in ultrasound imaging.
What Are the Next Steps?
The next steps for this research involve expanding the binary classification to a multiclass organ view identification. We also aim to delve into Active Learning in order to make the model more efficient in handling unlabeled instances, in addition to optimizing the model's performance and eventually deploy it in real-world clinical settings.
Paper Cited:
Self-Supervised Learning for Accurate Liver View Classification in Ultrasound Images With Minimal Labeled Data Abder-Rahman Ali, Anthony E. Samir, Peng Guo; Proceedings of the IEEE/CVF Conference on Computer Vision and Pattern Recognition (CVPR) Workshops, 2023, pp. 3086-3092
About the Massachusetts General Hospital
Massachusetts General Hospital, founded in 1811, is the original and largest teaching hospital of Harvard Medical School. The Mass General Research Institute conducts the largest hospital-based research program in the nation, with annual research operations of more than $1 billion and comprises more than 9,500 researchers working across more than 30 institutes, centers and departments. In July 2022, Mass General was named #8 in the U.S. News & World Report list of "America’s Best Hospitals." MGH is a founding member of the Mass General Brigham healthcare system.
Type
Centers and Departments
Topics
Check out the Mass General Research Institute blog
Bench Press highlights the groundbreaking research and boundary-pushing scientists working to improve human health and fight disease.
Support Research at Mass General
Your gift helps fund groundbreaking research aimed at understanding, treating and preventing human disease.